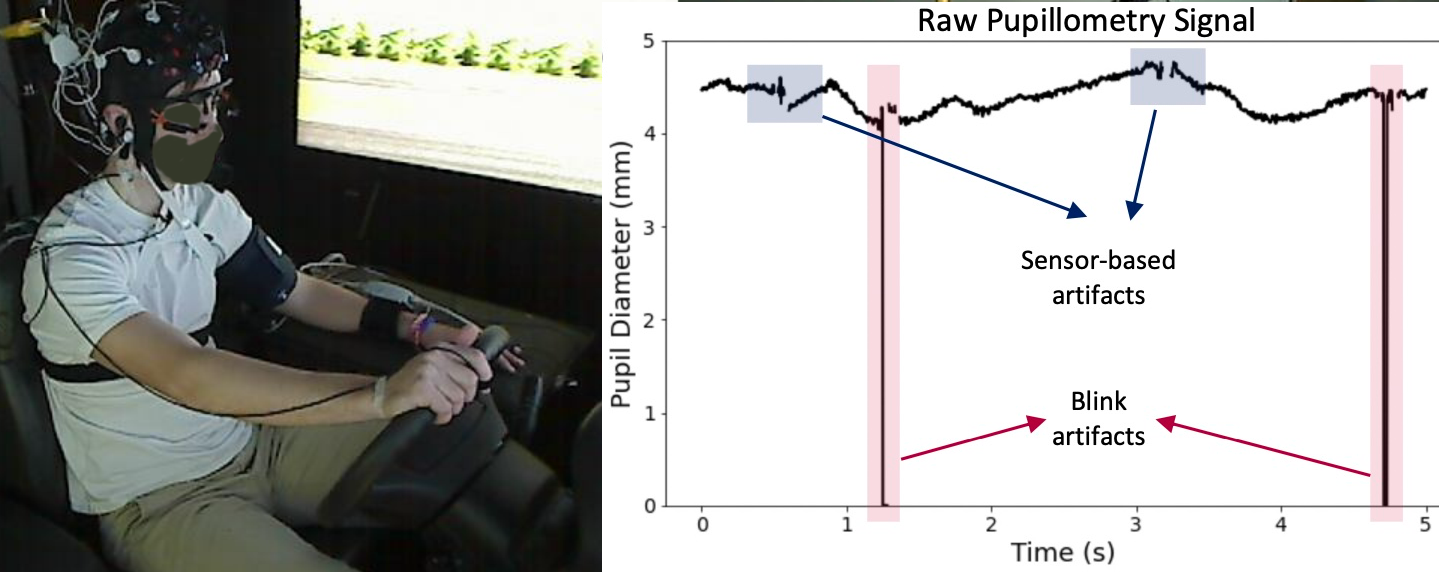
Assessing cognitive workload of human interactants in mixed initiative teams is a critical capability for autonomous interactive systems to enable adaptations that improve team performance. Yet, it is still unclear, due to diverg ing evidence, which sensing modality might work best for the determination of human workload. In this paper, we report results from an empirical study designed to answer this question by collecting eye gaze and electroencephalogram (EEG) data from human subjects performing an interactive multi-modal driving task. Different levels of cognitive workload were generated by introducing secondary tasks like dialogue, braking events, and tactile stimul ation in the course of driving. Our results show that pupil diameter is a more reliable indicator for workload prediction than EEG.
@inproceedings{aygunetal22icmi, title={Cognitive Workload Assessment via Eye Gaze and EEG in an Interactive Multi-Modal Driving Task}, author={Aygun, Ayca and Lyu, Boyang and Nguyen, Thuan and Haga, Zachary and Aeron, Shuchin and Scheutz, Matthias}, year={2022}, booktitle={Proceedings of the 2022 International Conference on Multimodal Interaction}, pages={337--348} url={https://hrilab.tufts.edu/publications/aygunetal22icmi.pdf} }